⚠️ 以下所有内容总结都来自于 大语言模型的能力,如有错误,仅供参考,谨慎使用
🔴 请注意:千万不要用于严肃的学术场景,只能用于论文阅读前的初筛!
💗 如果您觉得我们的项目对您有帮助 ChatPaperFree ,还请您给我们一些鼓励!⭐️ HuggingFace免费体验
2025-05-24 更新
Seeing through Satellite Images at Street Views
Authors:Ming Qian, Bin Tan, Qiuyu Wang, Xianwei Zheng, Hanjiang Xiong, Gui-Song Xia, Yujun Shen, Nan Xue
This paper studies the task of SatStreet-view synthesis, which aims to render photorealistic street-view panorama images and videos given any satellite image and specified camera positions or trajectories. We formulate to learn neural radiance field from paired images captured from satellite and street viewpoints, which comes to be a challenging learning problem due to the sparse-view natural and the extremely-large viewpoint changes between satellite and street-view images. We tackle the challenges based on a task-specific observation that street-view specific elements, including the sky and illumination effects are only visible in street-view panoramas, and present a novel approach Sat2Density++ to accomplish the goal of photo-realistic street-view panoramas rendering by modeling these street-view specific in neural networks. In the experiments, our method is testified on both urban and suburban scene datasets, demonstrating that Sat2Density++ is capable of rendering photorealistic street-view panoramas that are consistent across multiple views and faithful to the satellite image.
本文研究了SatStreet-view合成任务,该任务旨在根据任何卫星图像和指定的相机位置或轨迹呈现逼真的街道全景图像和视频。我们从卫星和街道观点捕获的配对图像中学习神经辐射场,这成为一个具有挑战性的学习问题,因为自然观点的稀疏性和卫星和街道观点图像之间视点变化极大。我们基于特定任务的观察来解决挑战,即街道视图特定元素,包括天空和照明效果仅在街道全景中可见,并提出了一种新方法Sat2Density++来完成渲染逼真街道全景的目标,通过在神经网络中建模这些街道视图特定元素。在实验部分中,我们的方法在都市和郊区场景数据集上得到了验证,证明了Sat2Density++能够渲染出跨多个视图一致且忠于卫星图像的逼真街道全景。
论文及项目相关链接
PDF Project page: https://qianmingduowan.github.io/sat2density-pp/, journal extension of ICCV 2023 conference paper ‘Sat2Density: Faithful Density Learning from Satellite-Ground Image Pairs’, submitted to TPAMI
Summary
本文研究了基于卫星图像和指定相机位置或轨迹生成逼真的街道视图全景图像和视频的任务。通过从卫星和街道视角捕获的配对图像学习神经辐射场,解决由于稀疏视图自然和卫星与街道视图图像之间极大视角变化所带来的挑战性问题。基于特定任务观察,提出一种新型方法Sat2Density++,利用神经网络建模街道视图特定元素(如天空和照明效果),实现逼真的街道视图全景渲染。实验证明,该方法在城市和郊区场景数据集上均表现优异,能够生成一致的多视角街道视图全景图像,并忠实于卫星图像。
Key Takeaways
- 该论文专注于从卫星图像渲染逼真的街道视图全景图像和视频的任务。
- 提出通过神经辐射场从配对图像中学习的方法,应对因视角变化带来的挑战。
- 基于街道视图特有的元素(如天空和照明),提出了一种新方法Sat2Density++。
- 实验证明该方法在城市和郊区场景数据集上表现良好。
- 该方法能够生成一致的多视角街道视图全景图像。
- 渲染的街道视图全景图像忠实于卫星图像。
点此查看论文截图
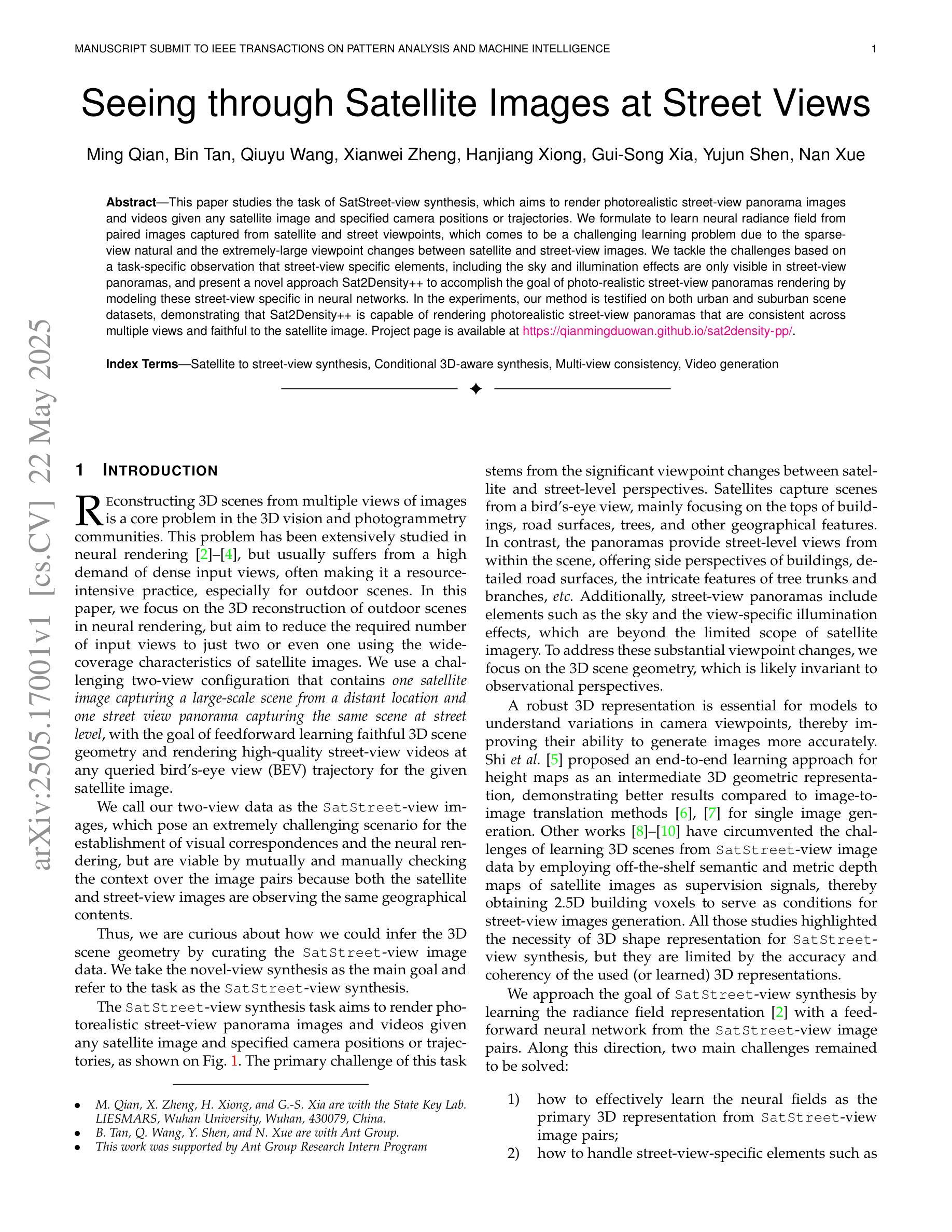
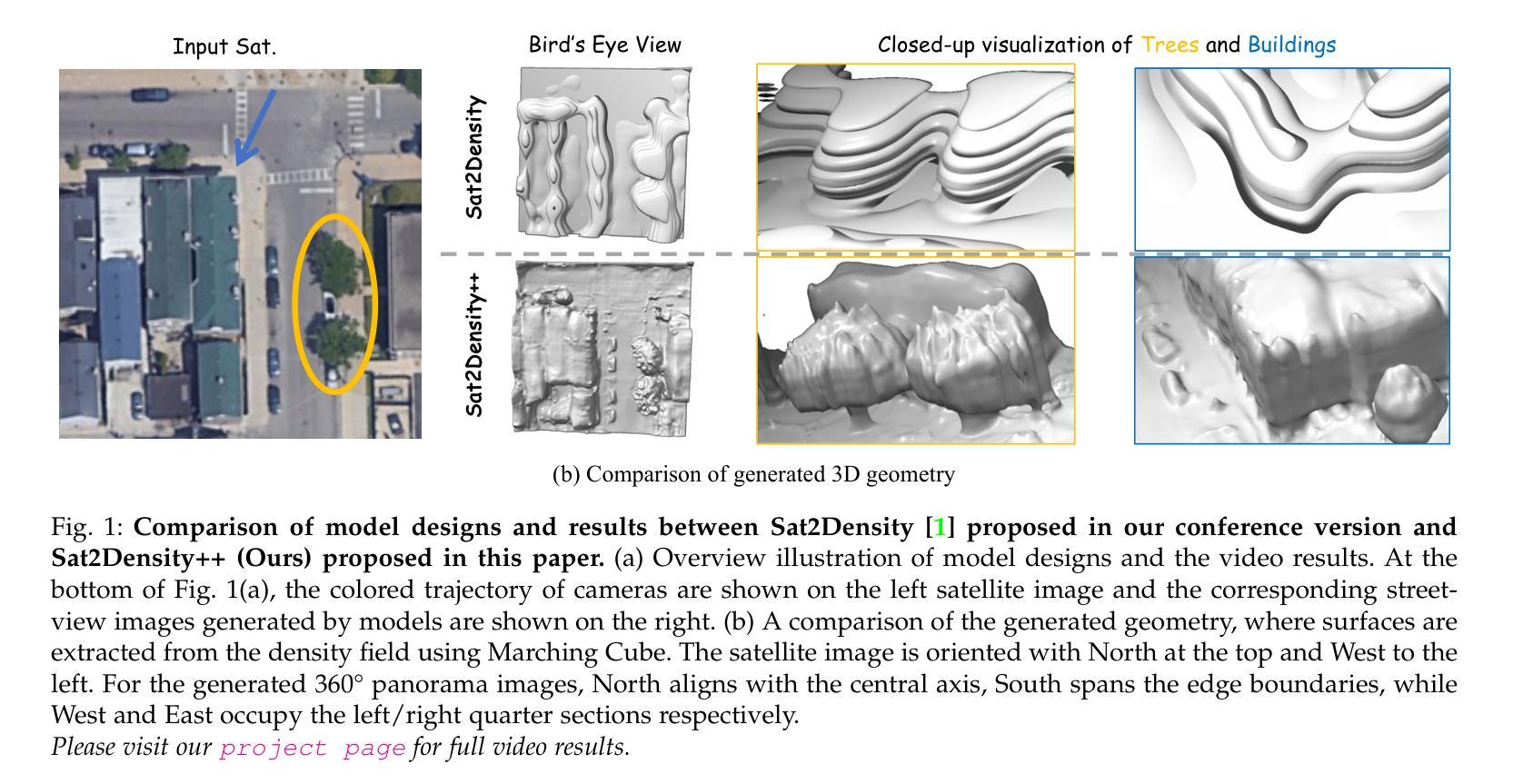
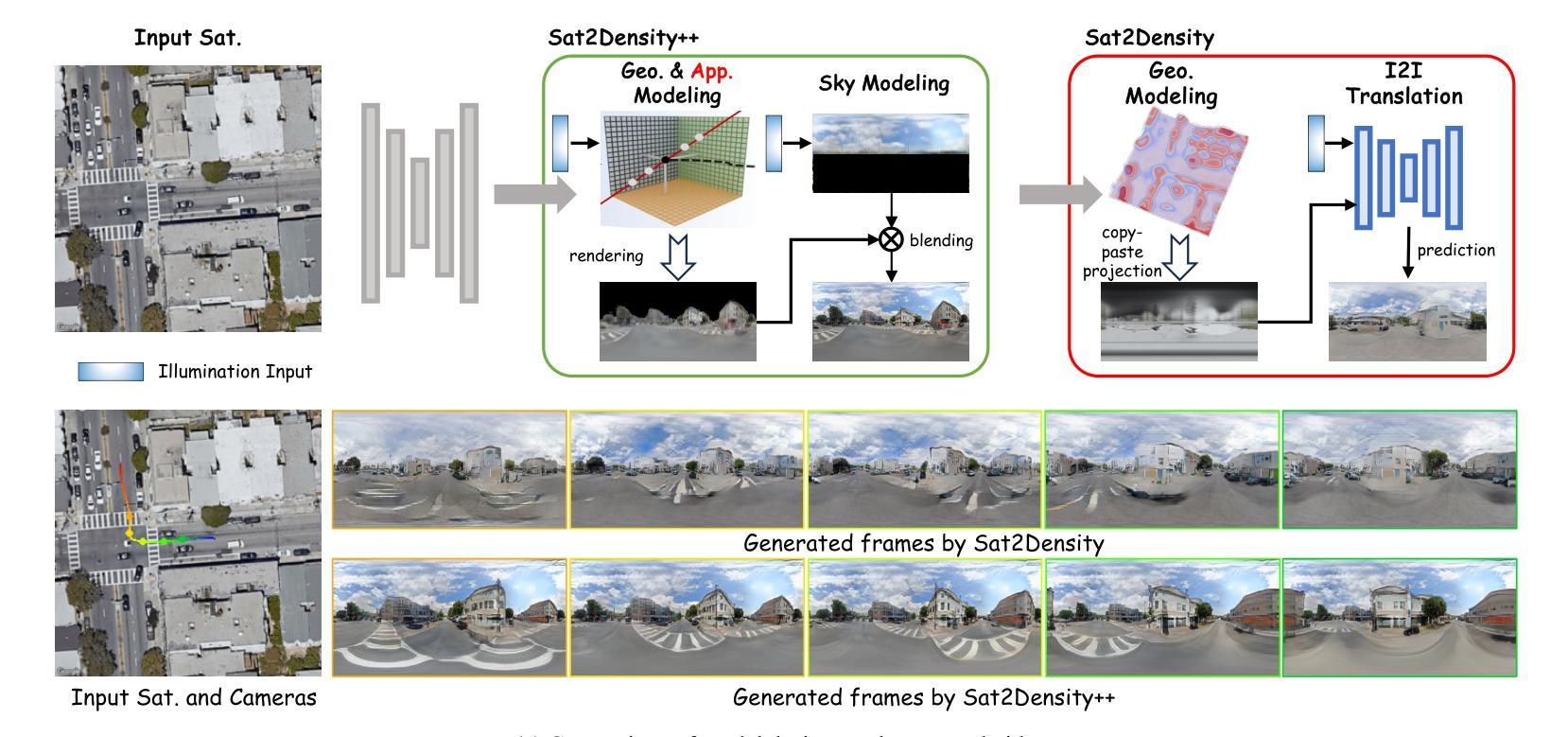
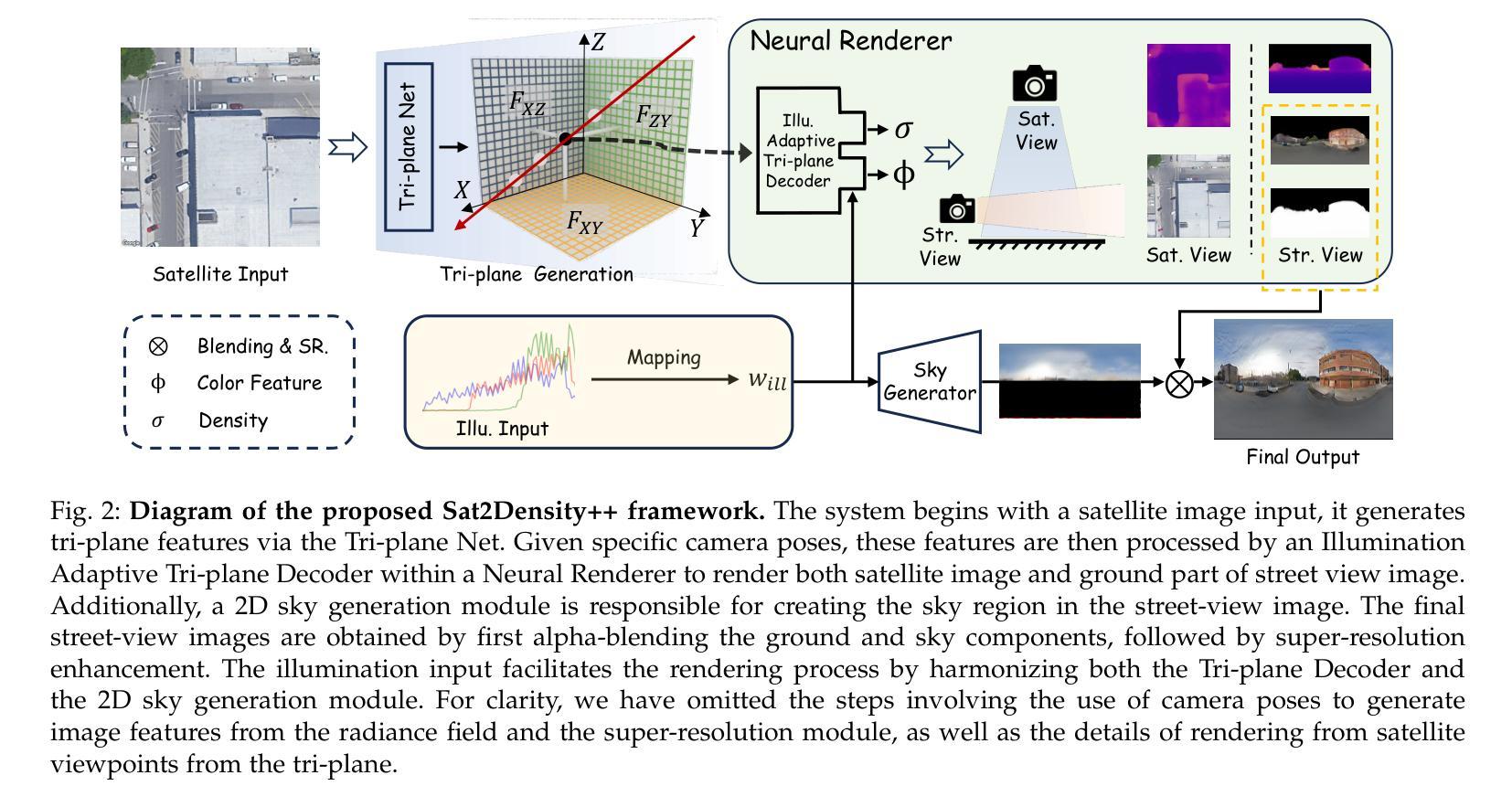
SuperPure: Efficient Purification of Localized and Distributed Adversarial Patches via Super-Resolution GAN Models
Authors:Hossein Khalili, Seongbin Park, Venkat Bollapragada, Nader Sehatbakhsh
As vision-based machine learning models are increasingly integrated into autonomous and cyber-physical systems, concerns about (physical) adversarial patch attacks are growing. While state-of-the-art defenses can achieve certified robustness with minimal impact on utility against highly-concentrated localized patch attacks, they fall short in two important areas: (i) State-of-the-art methods are vulnerable to low-noise distributed patches where perturbations are subtly dispersed to evade detection or masking, as shown recently by the DorPatch attack; (ii) Achieving high robustness with state-of-the-art methods is extremely time and resource-consuming, rendering them impractical for latency-sensitive applications in many cyber-physical systems. To address both robustness and latency issues, this paper proposes a new defense strategy for adversarial patch attacks called SuperPure. The key novelty is developing a pixel-wise masking scheme that is robust against both distributed and localized patches. The masking involves leveraging a GAN-based super-resolution scheme to gradually purify the image from adversarial patches. Our extensive evaluations using ImageNet and two standard classifiers, ResNet and EfficientNet, show that SuperPure advances the state-of-the-art in three major directions: (i) it improves the robustness against conventional localized patches by more than 20%, on average, while also improving top-1 clean accuracy by almost 10%; (ii) It achieves 58% robustness against distributed patch attacks (as opposed to 0% in state-of-the-art method, PatchCleanser); (iii) It decreases the defense end-to-end latency by over 98% compared to PatchCleanser. Our further analysis shows that SuperPure is robust against white-box attacks and different patch sizes. Our code is open-source.
随着基于视觉的机器学习模型越来越多地集成到自主和物理网络系统中,对(物理)对抗性补丁攻击的担忧也在增长。虽然最先进的防御手段可以在对高度集中局部补丁攻击时,以最小的影响实现认证的稳健性,但它们有两个不足之处:(i)最先进的防御方法容易受到低噪声分布补丁的攻击,这些补丁的扰动被微妙地分散开来以躲避检测或掩盖;(ii)使用最先进的防御方法实现高稳健性需要极其耗费时间和资源,这在许多物理网络系统的延迟敏感应用中并不实用。为了解决稳健性和延迟问题,本文提出了一种针对对抗补丁攻击的新防御策略,名为SuperPure。关键的创新之处在于开发了一种像素级的掩码方案,该方案对分布式和局部补丁都具有稳健性。掩码涉及利用基于生成对抗网络(GAN)的超分辨率方案来逐渐净化图像中的对抗补丁。我们在ImageNet上进行了大量评估,并使用ResNet和EfficientNet两种标准分类器,结果表明SuperPure在三个方面推动了前沿:(i)在常规局部补丁攻击方面,平均提高了超过20%的稳健性,同时提高了近10%的top-1清洁准确性;(ii)在对抗分布式补丁攻击方面达到了58%的稳健性(而现有的先进方法PatchCleanser为0%);(iii)与PatchCleanser相比,减少了超过98%的防御端到端延迟。我们的进一步分析表明,SuperPure对白色盒攻击和不同大小的补丁都具有稳健性。我们的代码是开源的。
论文及项目相关链接
Summary
针对基于视觉的机器学习模型在自主和物理系统中的集成应用,对抗性补丁攻击的问题日益突出。当前最先进的防御策略虽对高度集中、局部化的补丁攻击具有认证鲁棒性且对实用性影响较小,但在两方面仍有不足:一是易受低噪声分布式补丁攻击的影响;二是达到高鲁棒性的时间和资源消耗极大,对于许多物理系统中的延迟敏感应用来说并不实用。本文提出了一种新的对抗补丁攻击的防御策略——SuperPure,其关键创新之处在于开发了一种像素级的掩模方案,可抵抗分布式和局部补丁攻击。掩模利用基于GAN的超分辨率方案逐步净化图像中的对抗补丁。在ImageNet和两个标准分类器ResNet和EfficientNet的广泛评估中,SuperPure在三个方面推动了最新技术的前沿:提高了对常规局部补丁的鲁棒性;实现对分布式补丁攻击的鲁棒性;降低了防御端到端的延迟。
Key Takeaways
- 当前针对机器学习的对抗性补丁攻击的防御策略存在对低噪声分布式补丁攻击的脆弱性和高时间资源消耗的问题。
- SuperPure策略提出了一种像素级的掩模方案,该方案可抵抗分布式和局部补丁攻击。
- SuperPure利用基于GAN的超分辨率方案来逐步净化图像中的对抗补丁。
- SuperPure在ImageNet和两个标准分类器的评估中,提高了对常规局部补丁的鲁棒性,并且对分布式补丁攻击也具有鲁棒性。
- SuperPure相比现有方法,显著降低了防御策略的端到端延迟。
- SuperPure被证明可以抵抗白盒攻击和不同大小的补丁。
点此查看论文截图
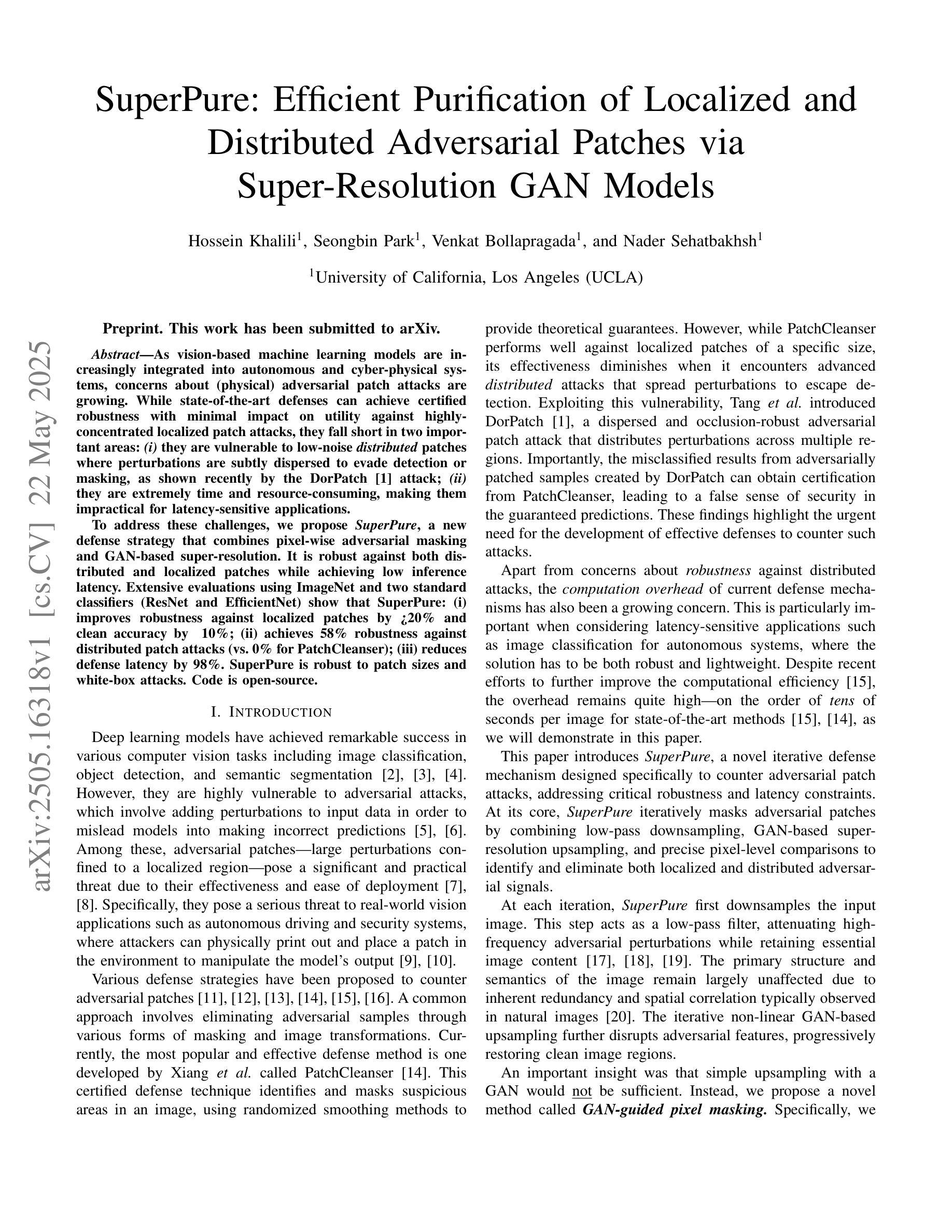
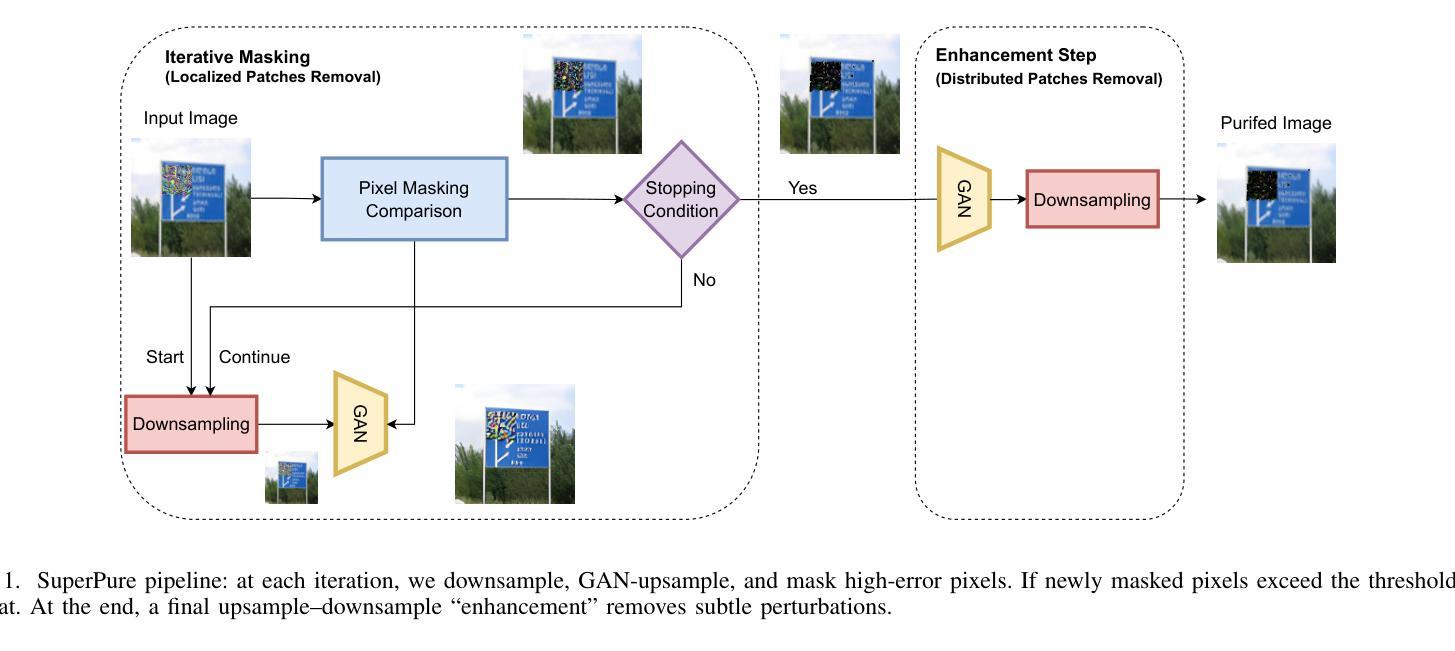
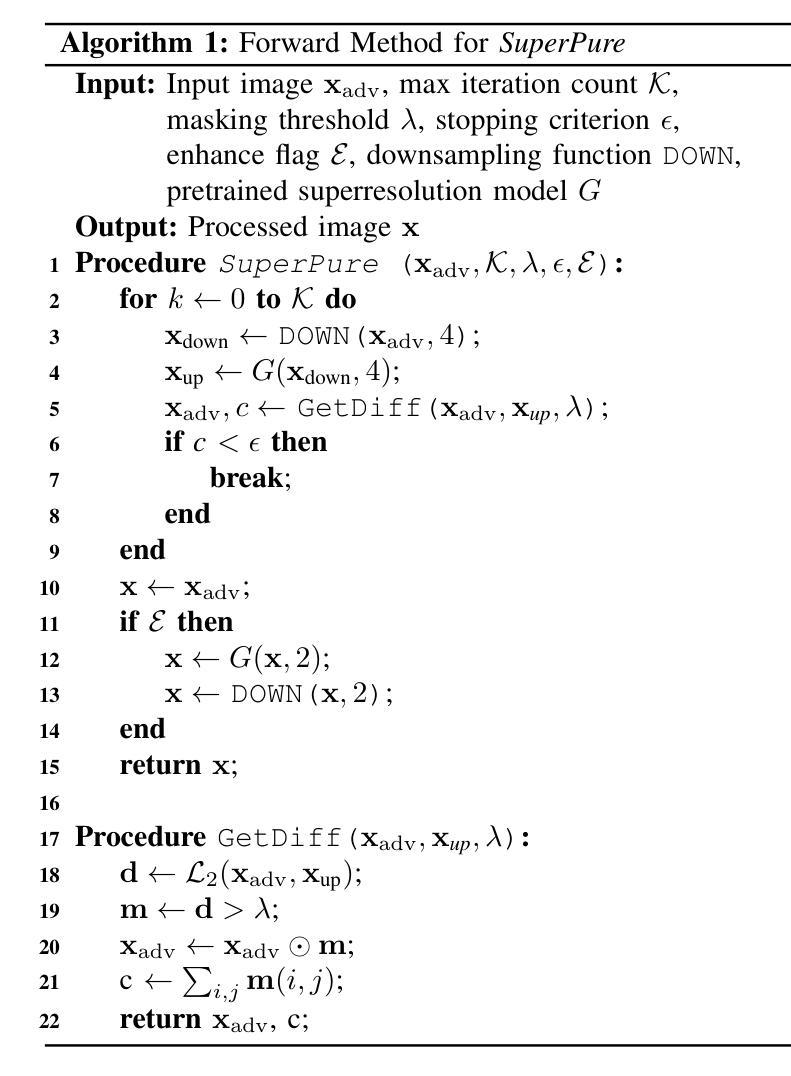
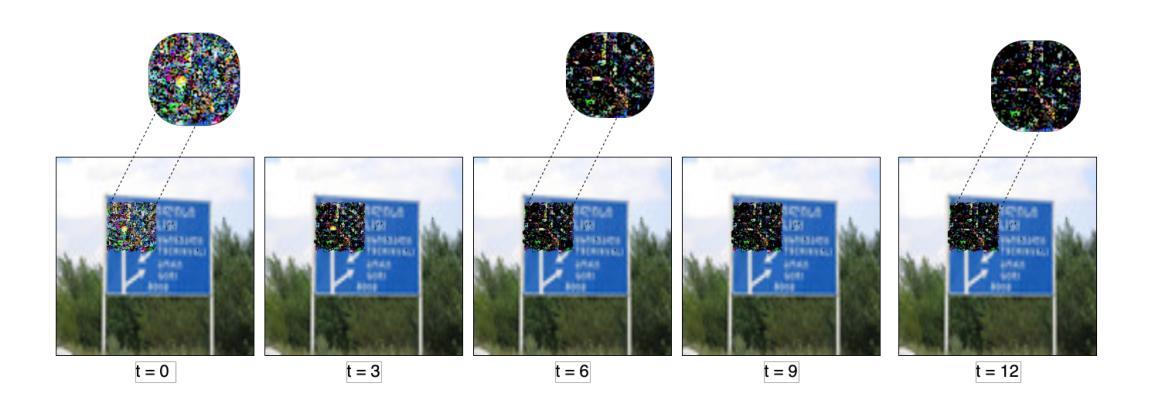
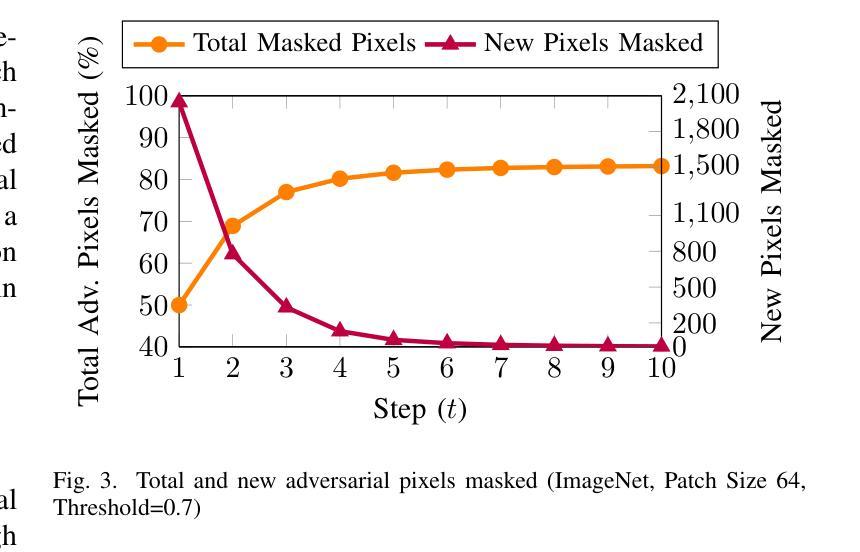