⚠️ 以下所有内容总结都来自于 大语言模型的能力,如有错误,仅供参考,谨慎使用
🔴 请注意:千万不要用于严肃的学术场景,只能用于论文阅读前的初筛!
💗 如果您觉得我们的项目对您有帮助 ChatPaperFree ,还请您给我们一些鼓励!⭐️ HuggingFace免费体验
2025-05-27 更新
Deep Video Discovery: Agentic Search with Tool Use for Long-form Video Understanding
Authors:Xiaoyi Zhang, Zhaoyang Jia, Zongyu Guo, Jiahao Li, Bin Li, Houqiang Li, Yan Lu
Long-form video understanding presents significant challenges due to extensive temporal-spatial complexity and the difficulty of question answering under such extended contexts. While Large Language Models (LLMs) have demonstrated considerable advancements in video analysis capabilities and long context handling, they continue to exhibit limitations when processing information-dense hour-long videos. To overcome such limitations, we propose the Deep Video Discovery agent to leverage an agentic search strategy over segmented video clips. Different from previous video agents manually designing a rigid workflow, our approach emphasizes the autonomous nature of agents. By providing a set of search-centric tools on multi-granular video database, our DVD agent leverages the advanced reasoning capability of LLM to plan on its current observation state, strategically selects tools, formulates appropriate parameters for actions, and iteratively refines its internal reasoning in light of the gathered information. We perform comprehensive evaluation on multiple long video understanding benchmarks that demonstrates the advantage of the entire system design. Our DVD agent achieves SOTA performance, significantly surpassing prior works by a large margin on the challenging LVBench dataset. Comprehensive ablation studies and in-depth tool analyses are also provided, yielding insights to further advance intelligent agents tailored for long-form video understanding tasks. The code will be released later.
长视频理解面临着巨大的挑战,这主要是因为存在巨大的时空复杂性和在这样扩展的上下文中进行问答的困难。虽然大型语言模型(LLM)在视频分析能力和长文本处理能力方面取得了显著的进步,但在处理信息密集的一小时长的视频时仍存在局限性。为了克服这些局限性,我们提出了Deep Video Discovery(DVD)代理,利用分段视频剪辑上的智能搜索策略。不同于之前手动设计僵化工作流程的视频代理,我们的方法侧重于代理的自主性。通过提供多粒度视频数据库上一系列搜索中心工具,我们的DVD代理利用LLM的高级推理能力来规划其当前观察状态,有选择性地灵活选取工具、为行动制定适当参数并根据获取的信息不断精炼其内部推理。我们对多个长视频理解基准进行了全面的评估,展示了整个系统设计的优势。我们的DVD代理实现了最先进的性能,在具有挑战性的LVBench数据集上大幅超越了以前的工作。我们还提供了全面的消融研究和深入的工具分析,为针对长视频理解任务量身定制的智能代理的进一步发展提供了见解。代码将在稍后发布。
论文及项目相关链接
PDF Under review
Summary
长视频理解面临巨大的挑战,如复杂的时空特性和在较长语境下进行问答的困难。虽然大型语言模型(LLM)在视频分析能力和长语境处理方面取得了显著的进步,但在处理信息密集的小时长的视频时仍表现出局限性。为了克服这些局限性,我们提出了深度视频发现代理(DVD agent),采用基于代理的搜索策略来处理分段视频剪辑。我们的方法与以往手动设计刚性工作流程的视频代理不同,更加强调代理的自主性。通过在多粒度视频数据库上提供一系列搜索中心工具,DVD代理利用LLM的高级推理能力来规划其当前观察状态,灵活选择工具,为行动制定适当参数,并根据获取的信息迭代优化其内部推理。我们在多个长视频理解基准测试上对DVD代理进行了全面评估,证明了整个系统设计的优势。在具有挑战性的LVBench数据集上,我们的DVD代理取得了最先进的性能表现,全面超越了以前的工作。同时提供了全面的消融研究和深入的工具分析,以推动针对长视频理解任务的智能代理的进一步发展。相关代码即将发布。
Key Takeaways
- 长视频理解面临挑战,包括处理大量信息和在不同语境下进行问答。
- 大型语言模型(LLM)在视频分析和处理长语境信息方面已取得进步,但对信息密集的长视频仍有局限性。
- 提出的深度视频发现代理(DVD agent)利用自主搜索策略处理分段视频剪辑。
- DVD代理强调自主性,通过选择工具、制定行动参数和优化内部推理来克服局限性。
- 在多个长视频理解基准测试中评估DVD代理,证明其性能优越。
- DVD代理在具有挑战性的LVBench数据集上取得显著成果,超越先前的工作。
点此查看论文截图
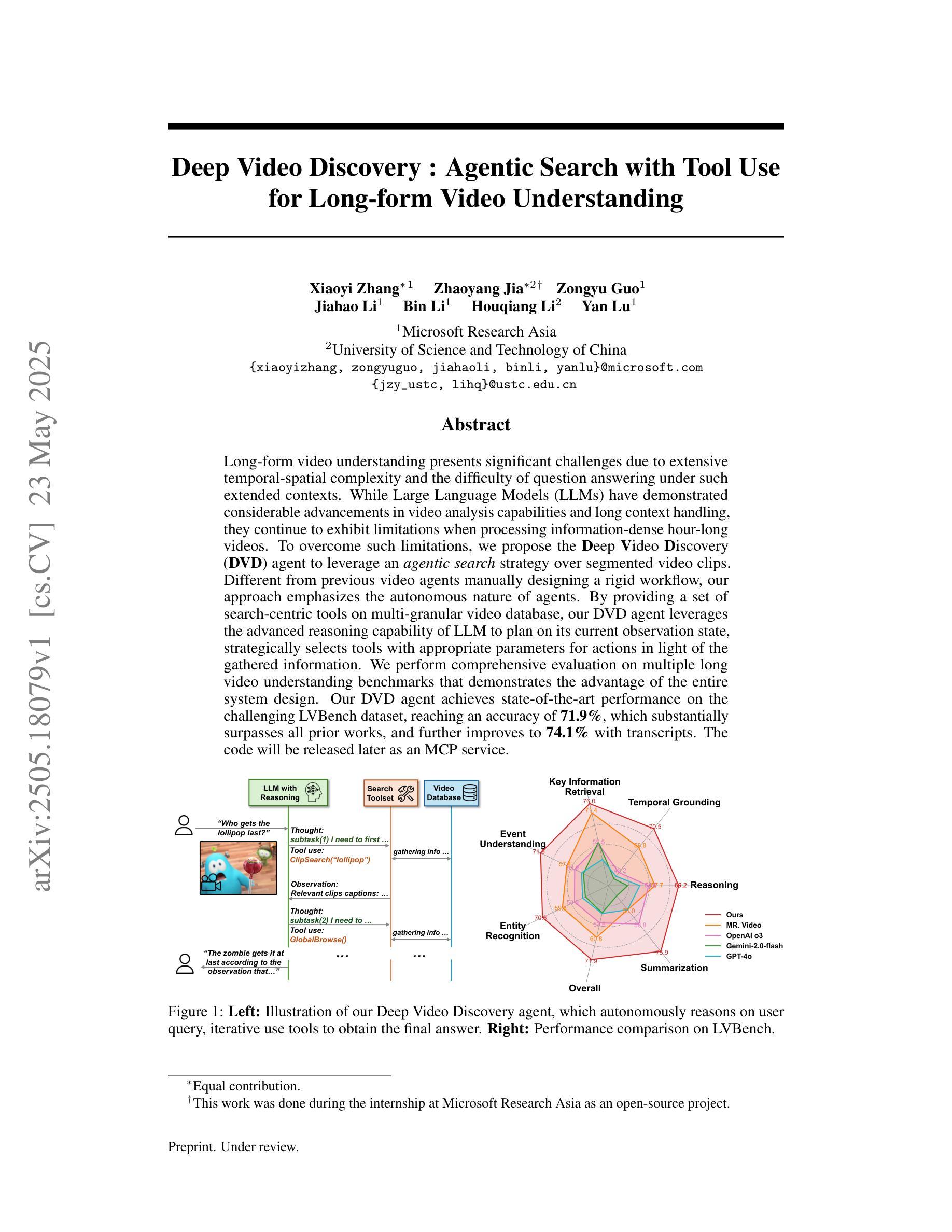
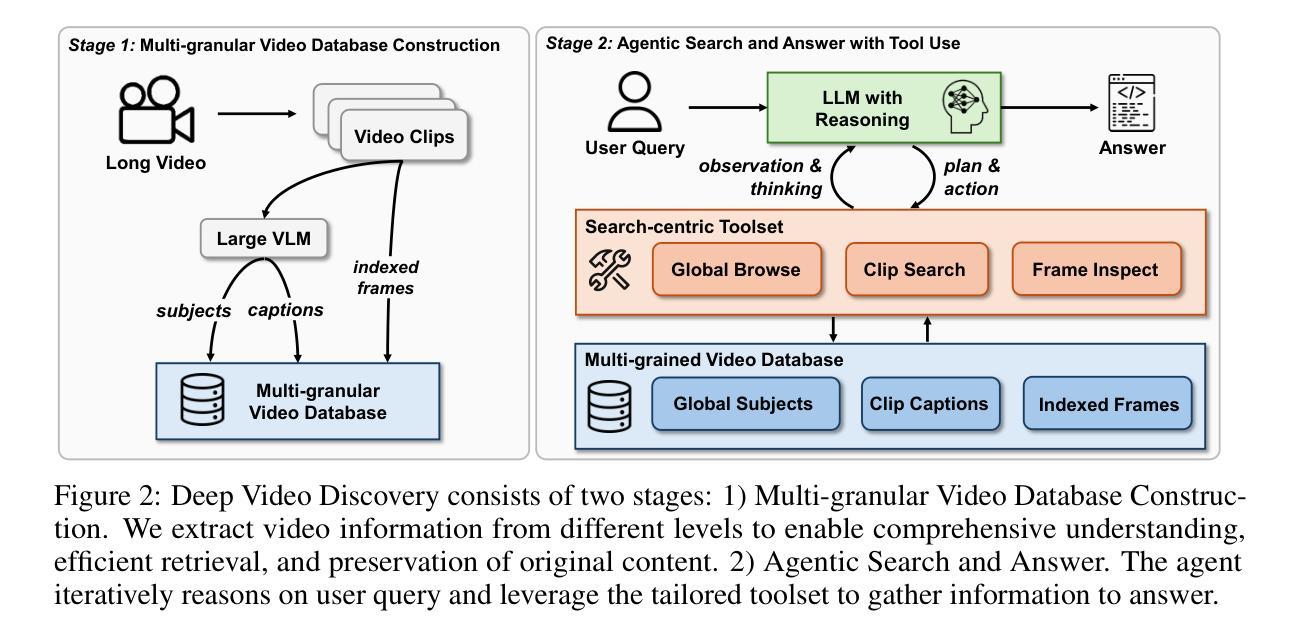
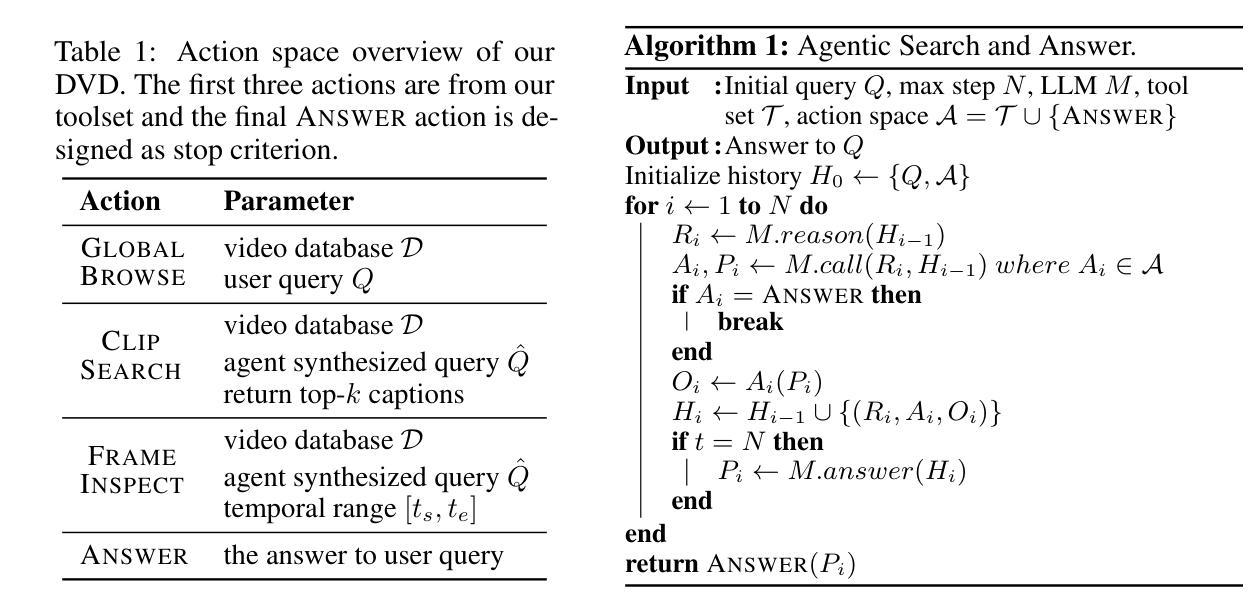