⚠️ 以下所有内容总结都来自于 大语言模型的能力,如有错误,仅供参考,谨慎使用
🔴 请注意:千万不要用于严肃的学术场景,只能用于论文阅读前的初筛!
💗 如果您觉得我们的项目对您有帮助 ChatPaperFree ,还请您给我们一些鼓励!⭐️ HuggingFace免费体验
2025-05-28 更新
Towards Generalized Proactive Defense against Face Swappingwith Contour-Hybrid Watermark
Authors:Ruiyang Xia, Dawei Zhou, Decheng Liu, Lin Yuan, Jie Li, Nannan Wang, Xinbo Gao
Face swapping, recognized as a privacy and security concern, has prompted considerable defensive research. With the advancements in AI-generated content, the discrepancies between the real and swapped faces have become nuanced. Considering the difficulty of forged traces detection, we shift the focus to the face swapping purpose and proactively embed elaborate watermarks against unknown face swapping techniques. Given that the constant purpose is to swap the original face identity while preserving the background, we concentrate on the regions surrounding the face to ensure robust watermark generation, while embedding the contour texture and face identity information to achieve progressive image determination. The watermark is located in the facial contour and contains hybrid messages, dubbed the contour-hybrid watermark (CMark). Our approach generalizes face swapping detection without requiring any swapping techniques during training and the storage of large-scale messages in advance. Experiments conducted across 8 face swapping techniques demonstrate the superiority of our approach compared with state-of-the-art passive and proactive detectors while achieving a favorable balance between the image quality and watermark robustness.
人脸替换技术被视为隐私和安全问题而受到广泛关注,并引发了大量防御性研究。随着人工智能生成内容的进步,真实人脸和替换人脸之间的差异变得微妙。考虑到难以检测伪造痕迹,我们将重点转向人脸替换的目的,并针对未知的人脸替换技术主动嵌入精细的水印。考虑到始终的目的是在保留背景的同时替换原始人脸身份,我们将重点关注人脸周围的区域,以确保生成稳健的水印,同时嵌入轮廓纹理和人脸身份信息以实现渐进的图像确定。水印位于面部轮廓上,包含混合信息,被称为轮廓混合水印(CMark)。我们的方法通过对面部轮廓的通用处理来检测人脸替换,无需在训练期间使用任何替换技术,也不需要预先存储大规模信息。在8种人脸替换技术上进行实验表明,与最新被动和主动检测器相比,我们的方法具有优势,同时在图像质量和水印稳健性之间取得了良好的平衡。
论文及项目相关链接
PDF 16 pages, 11 figures, under review
摘要
随着人工智能生成内容的进步,面部替换技术引发了隐私和安全问题。研究重点已转向面部替换的目的,并主动嵌入针对未知面部替换技术的复杂水印。该研究侧重于在保留背景的同时,对面部身份进行替换,因此在面部轮廓周围确保稳健的水印生成,同时嵌入轮廓纹理和面部身份信息以实现渐进的图像识别。水印位于面部轮廓上并包含混合信息,称为轮廓混合水印(CMark)。我们的方法无需在训练期间使用任何替换技术即可通用化面部替换检测,并且在事先存储大规模信息的同时实现了图像质量和水印稳健性的良好平衡。在八种面部替换技术上的实验表明,我们的方法相较于最新的被动和主动检测器具有优势。
关键见解
- 研究关注面部替换的目的,并主动嵌入复杂水印来应对未知面部替换技术。
- 水印嵌入面部轮廓周围以确保稳健性,同时嵌入轮廓纹理和面部身份信息。
- 提出一种名为轮廓混合水印(CMark)的水印技术。
- 方法可通用化面部替换检测,无需在训练期间使用任何替换技术。
- 在图像质量和水印稳健性之间实现了良好的平衡。
- 在八种面部替换技术上的实验证明,该方法相较于现有技术具有优势。
点此查看论文截图
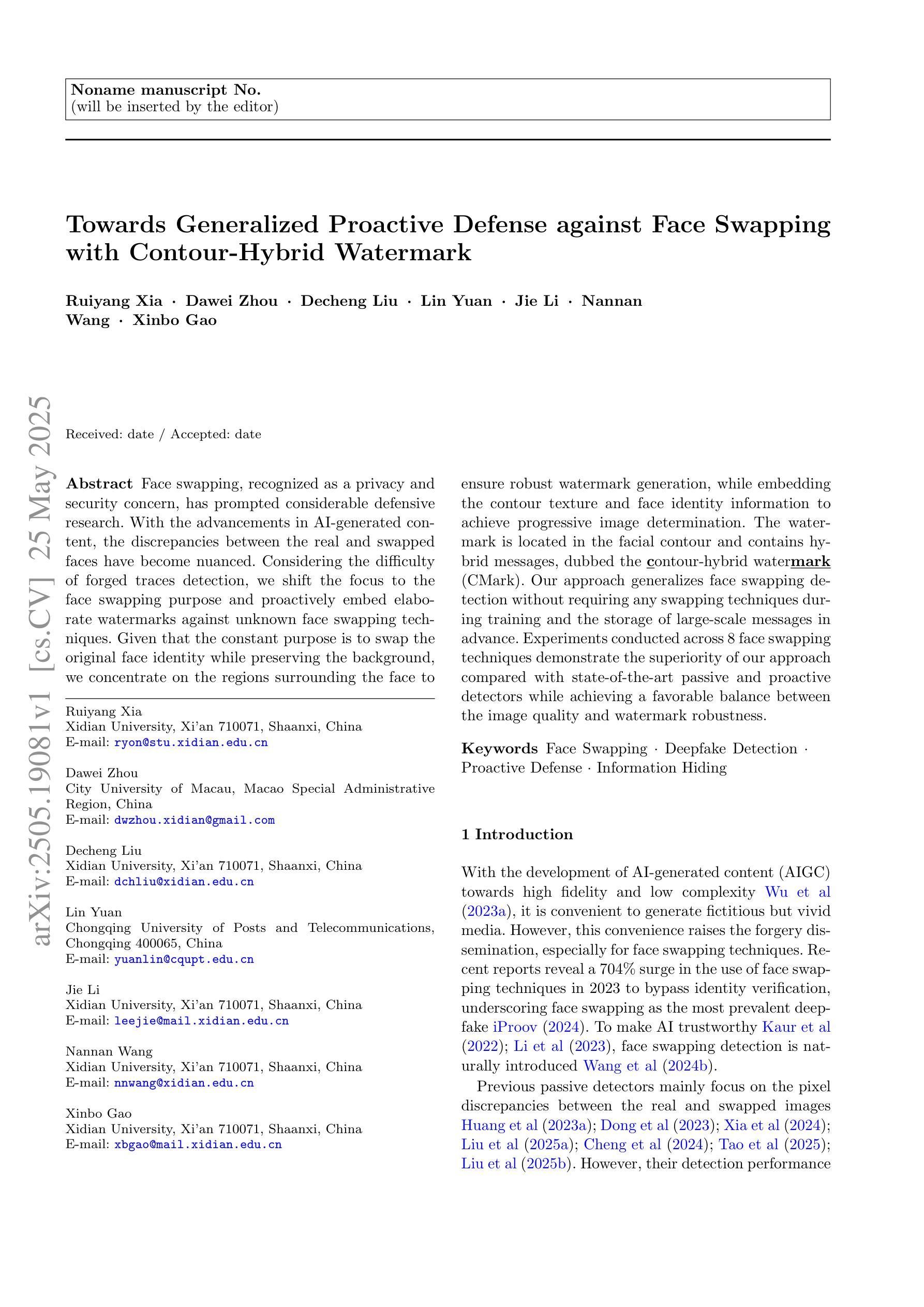
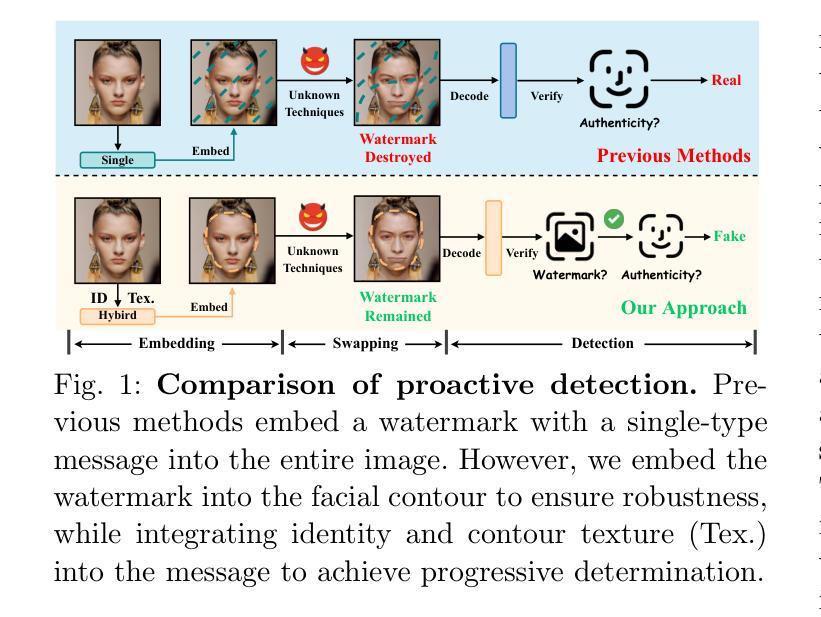
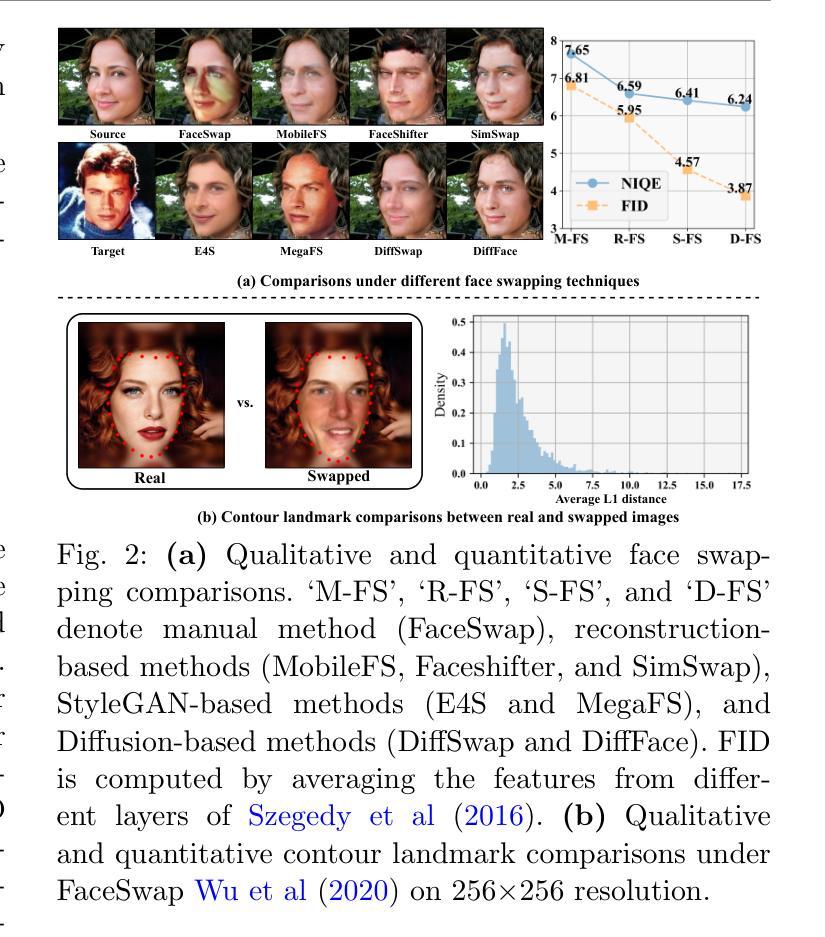
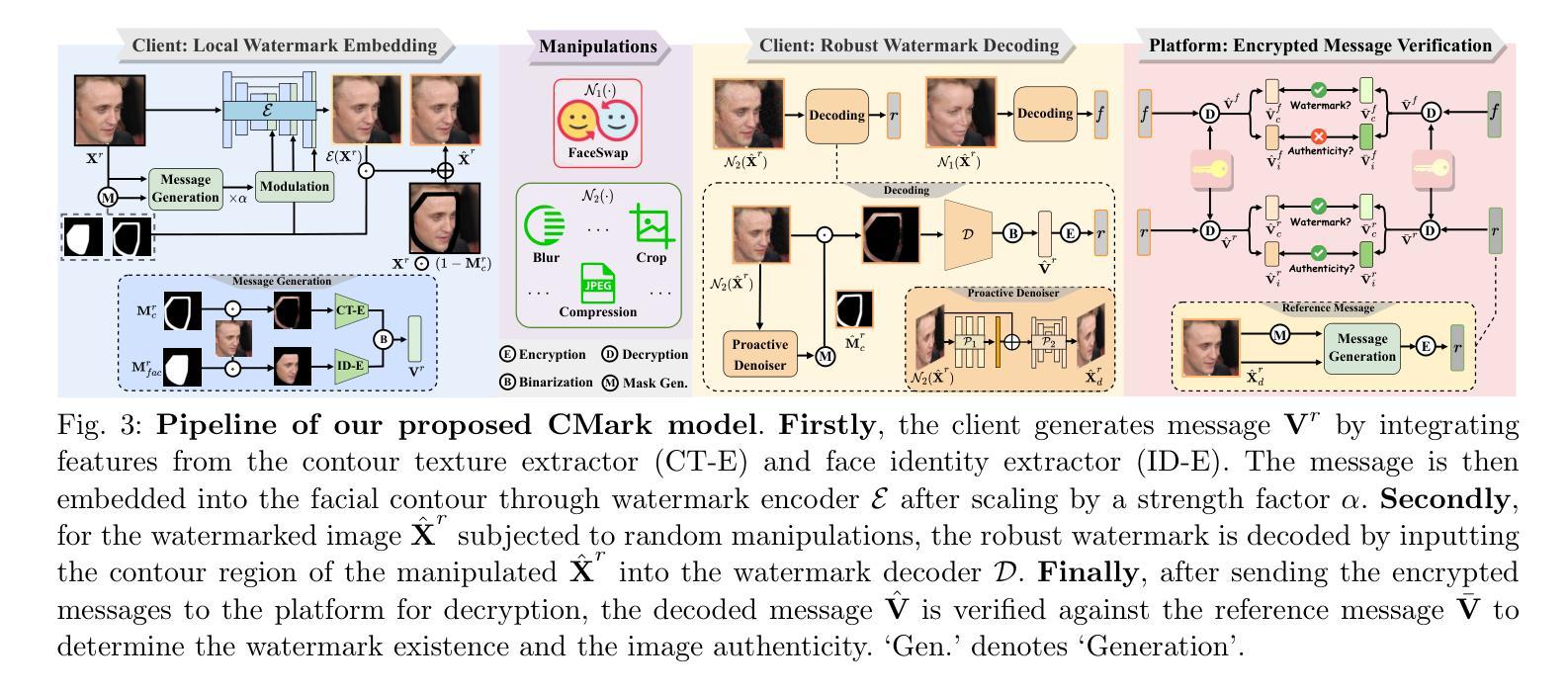
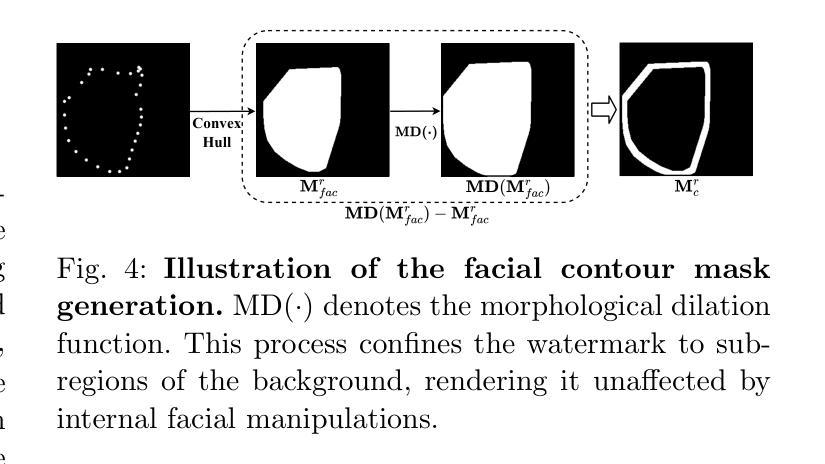
WaveGuard: Robust Deepfake Detection and Source Tracing via Dual-Tree Complex Wavelet and Graph Neural Networks
Authors:Ziyuan He, Zhiqing Guo, Liejun Wang, Gaobo Yang, Yunfeng Diao, Dan Ma
Deepfake technology poses increasing risks such as privacy invasion and identity theft. To address these threats, we propose WaveGuard, a proactive watermarking framework that enhances robustness and imperceptibility via frequency-domain embedding and graph-based structural consistency. Specifically, we embed watermarks into high-frequency sub-bands using Dual-Tree Complex Wavelet Transform (DT-CWT) and employ a Structural Consistency Graph Neural Network (SC-GNN) to preserve visual quality. We also design an attention module to refine embedding precision. Experimental results on face swap and reenactment tasks demonstrate that WaveGuard outperforms state-of-the-art methods in both robustness and visual quality. Code is available at https://github.com/vpsg-research/WaveGuard.
深度伪造技术带来了日益严重的风险,如侵犯隐私和身份盗窃。为了解决这些威胁,我们提出了WaveGuard,这是一个主动的水印框架,通过频域嵌入和基于图的结构一致性增强稳健性和隐蔽性。具体来说,我们使用双树复数小波变换(DT-CWT)将水印嵌入高频子带,并采用结构一致性图神经网络(SC-GNN)来保持视觉质量。我们还设计了一个注意力模块来提高嵌入精度。在换脸和面部再现任务上的实验结果表明,WaveGuard在稳健性和视觉质量方面都优于最先进的方法。代码可在https://github.com/vpsg-research/WaveGuard找到。
论文及项目相关链接
PDF 12 pages, 6 figures, 5 tables
Summary
针对Deepfake技术带来的隐私入侵和身份盗窃等风险,提出了WaveGuard主动水印框架。该框架通过频域嵌入和基于图的结构一致性增强稳健性和隐蔽性。在高频子带中嵌入水印,并利用结构一致性图神经网络保留视觉质量。同时,设计了一个注意力模块来提高嵌入精度。在面部交换和重新演绎任务上的实验结果表明,WaveGuard在稳健性和视觉质量方面优于现有技术。
Key Takeaways
- Deepfake技术存在隐私入侵和身份盗窃等风险。
- WaveGuard是一个主动水印框架,旨在解决这些问题。
- 该框架利用频域嵌入技术,通过Dual-Tree Complex Wavelet Transform (DT-CWT)嵌入水印。
- WaveGuard利用结构一致性图神经网络(SC-GNN)保持视觉质量。
- 通过注意力模块提高嵌入精度。
- 实验结果表明WaveGuard在面部交换和重新演绎任务方面表现优异。
点此查看论文截图
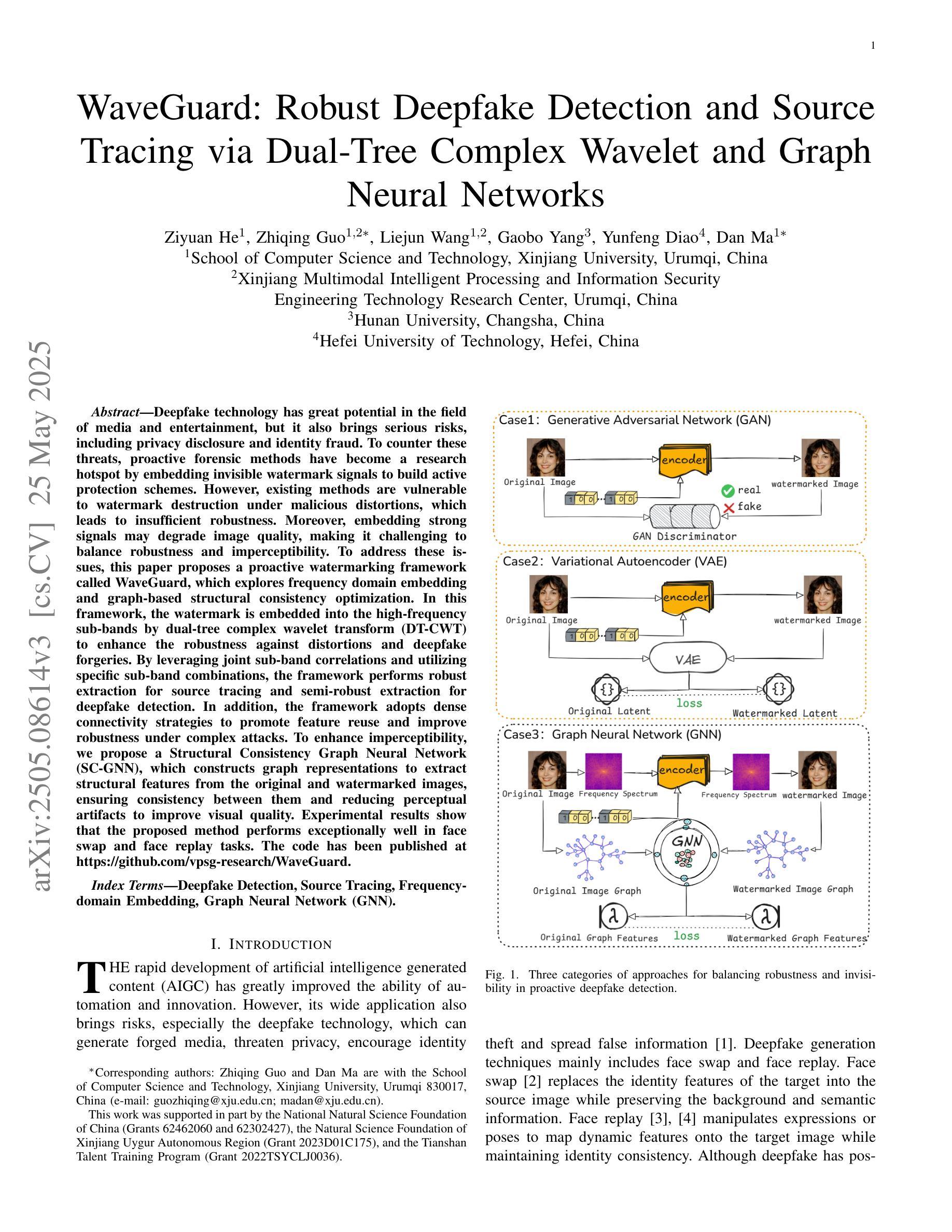
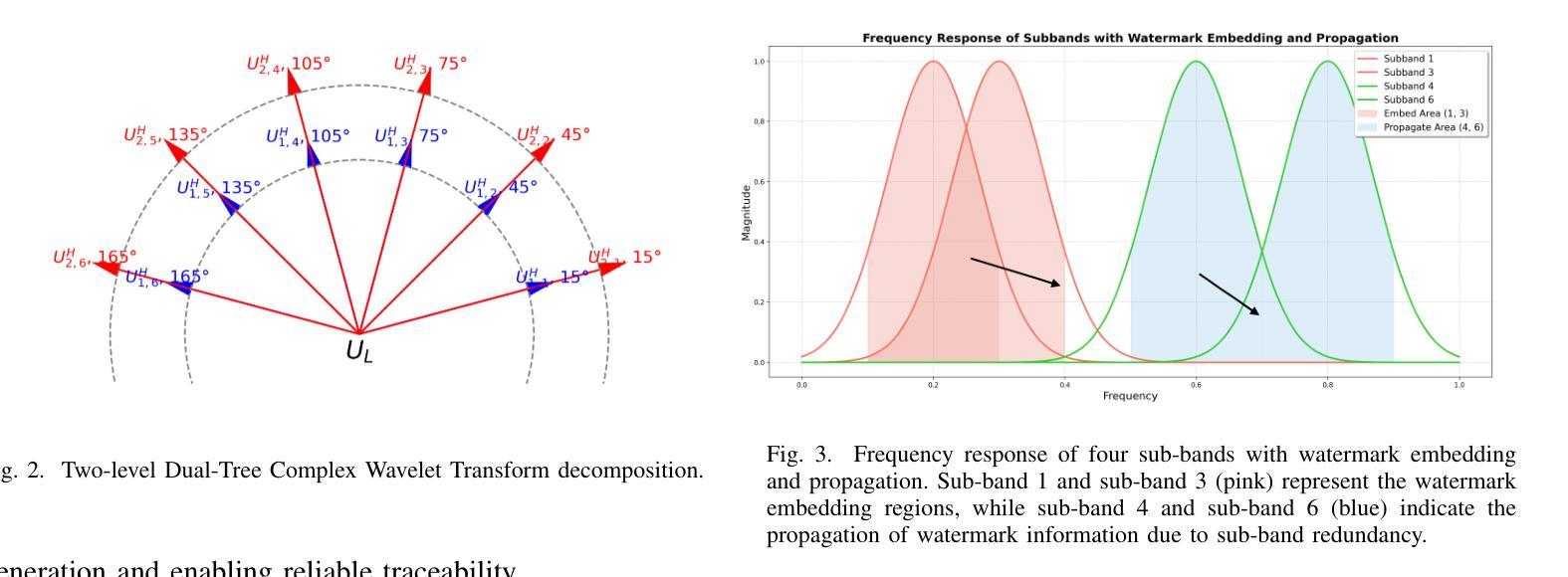
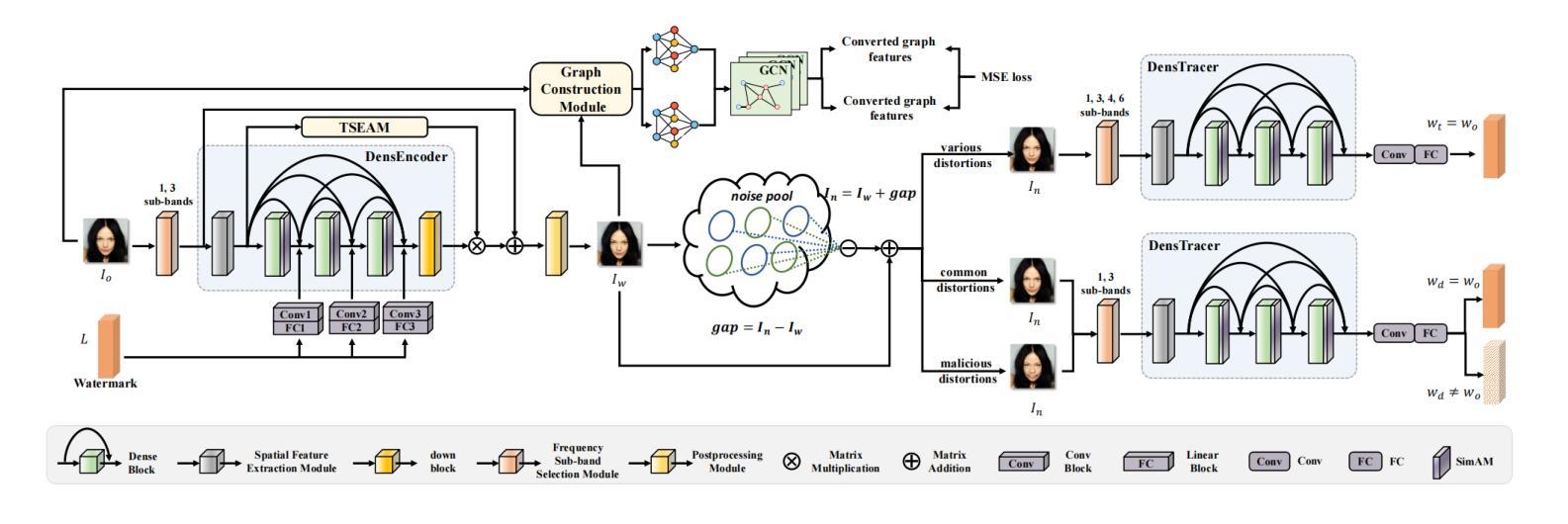